CU-UTMO Columbia University
To estimate future trends in uterine cancer incidence and mortality, and identify optimal screening, detection, and treatment strategies to reduce the burden of this disease.
Contact: Jason Wright jw2459@cumc.columbia.edu
Summary
Both the incidence and mortality of uterine cancer is increasing in the United States, and modeling is needed to forecast future trends of the disease, and identify optimal screening, detection, and treatment modalities in women at varying levels of risk. Therefore, we developed a natural history model for uterine cancer calibrated to the population-based incidence and mortality of the disease.
The Columbia University Uterine Cancer Model (CU-UTMO) is an individual-level stochastic state transition microsimulation that includes endometrioid, non-endometrioid, and sarcoma histologies. The cycle length for this model is one month. Simulated individuals may transition between health states as illustrated in Figure 1. The model includes a potential precursor uterine cancer: endometrial intraepithelial neoplasia (EIN). The model assumes that body mass index (BMI) impacts the development of EIN and uterine cancer as indicated by the red transitions between health states in Figure 1. Simulated individuals may transition to death from non-uterine cancer causes at any point and those with uterine cancer face a competing risk of uterine cancer death. The transition probabilities vary by single year age, and 10-year birth cohort categories, starting with 1910-1919.
Structure of CU-UTMO
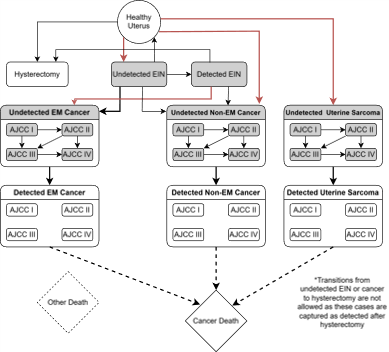
Figure 1: Structure of CU-UTMO *EIN: Endometrial Intraepithelial Neoplasia
We identified optimal data sources for our model inputs and calibration targets. Incidence data from SEER 18, stratified by age, AJCC stage, race/ethnicity and birth cohort was the primary calibration target. We used SEER survival data up to 10 years post diagnosis to generate hazard functions for death from uterine cancer. We used CDC Wonder data for all-cause mortality, stratified by age, race/ethnicity and birth cohort. We used NHANES data for hysterectomy and obesity rates as inputs for the model.
Using this model, we will be able to examine the impact of changing risk factor profiles on future trends in uterine cancer incidence and mortality. Further, we will be able to evaluate the impact of primary and secondary prevention strategies, and new treatment regimens, at the population-level, and within high-risk groups, to support national guidelines and policies to reduce the burden of uterine cancer.
Bladder models
- Kystis (Brown) Brown
- COBRAS (Ottawa) Ottawa
- SCOUT (NYU) NYU
Bladder Model Comparison Grid (PDF, 145 KB)
See all Comparison Grids & Profiles (Includes historical versions)
Lung models
- BCCRI-LunCan (BCCRI)
- BCCRI-Smoking (BCCRI)
- LCOS (Stanford)
- LCPM (MGH)
- MISCAN-Lung (Erasmus)
- SimSmoke (Georgetown)
- Smoking-Lung Cancer (Georgetown)
- MULU (Mount Sinai)
- ENGAGE (MDACC)
- YLCM (Yale)
- OncoSim-Lung (CPAC-StatCan)
- LMO (FHCC) (Historical)
Lung Model Comparison Grid (PDF, 161 KB)
See all Comparison Grids & Profiles (Includes historical versions)